Article
The path to AI-driven autonomous telecoms networks
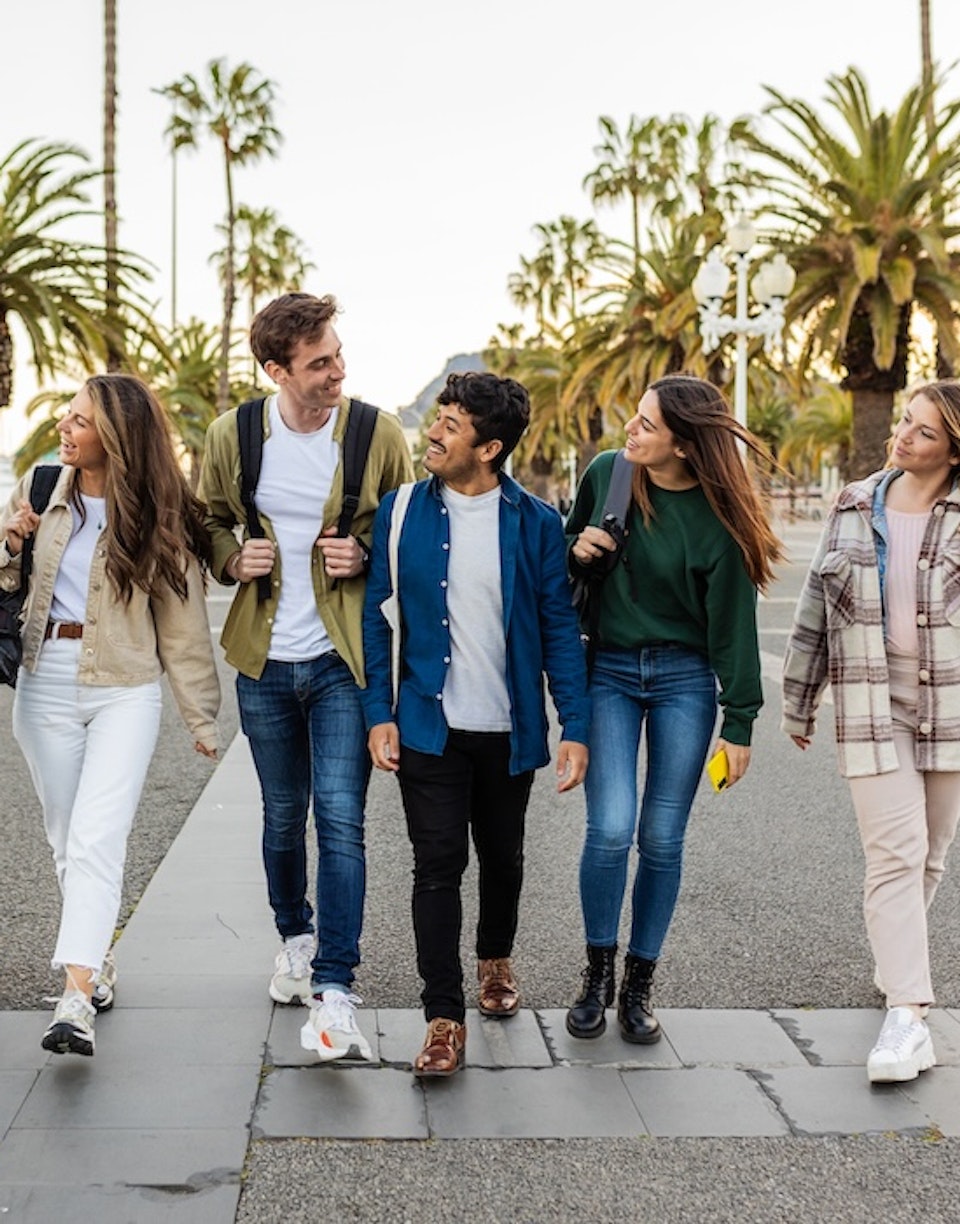
The evolution towards autonomous networks is key to improving operations and maintenance, allowing telco operators to tackle the current challenges in the sector
Main challenges for telecommunications operators
Telecommunications operators face multiple challenges: the continuous increase in demand, which stresses operational processes; the complexity of networks due to new technologies; intense competition requiring differentiation; the need for high investment to offer competitive services; high energy costs, which can represent between 30% and 40% of total operational costs; and increasingly sophisticated threats that heighten cybersecurity risks.
To address these challenges, it is crucial to improve business aspects such as go-to-market strategies, operational efficiency, customer experience, revenue growth, and energy efficiency.
The promise of autonomous networks
The new paradigm is the autonomous network, promising significant improvements in operations and maintenance. The evolution towards this paradigm involves moving from networks operated by humans assisted by systems to networks autonomously operated by systems, with minimal human intervention.
These networks are designed to self-configure, self-repair, and self-optimize with little or no human intervention. For example, they can automatically detect and diagnose performance issues, reroute traffic in case of failures, and predict potential problems. This level of self-regulation leads to greater efficiency, performance, and reliability, while also reducing costs and minimizing the chances of human error.
With the growing complexity of today's networks, autonomous network processes must include solutions that incorporate artificial intelligence to make decisions in changing environments without human intervention.
TM Forum and the regulatory framework for autonomous networks
TM Forum, a leading organization in the sector, has established standards and frameworks that guide companies in the implementation and management of autonomous networks. Their guidelines facilitate the adoption of advanced AI and machine learning technologies, promoting interoperability and operational efficiency in increasingly complex networks.
TM Forum has defined six maturity levels to measure the degree of network autonomy. Most Tier-1 operators are already setting goals to reach medium-high maturity levels in the medium term. A clear example in Spain is Telefónica, which has set a goal to reach a maturity level 4 by 2025.
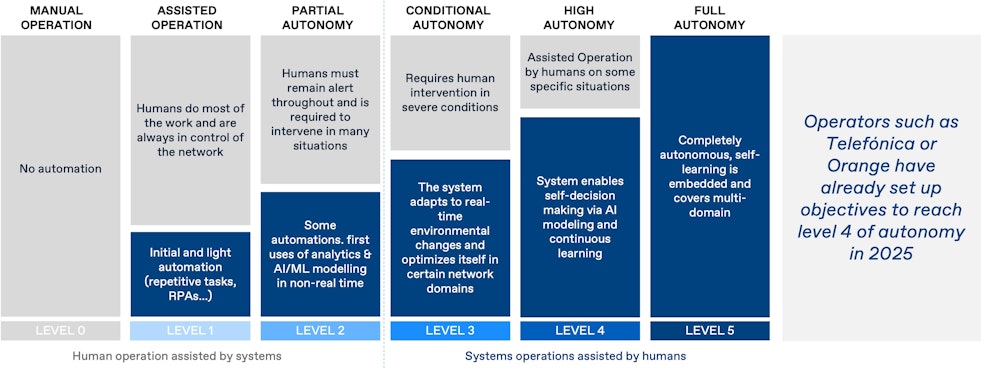
Key technologies for the evolution of autonomous networks
Advancing towards autonomous networks would not be possible without the intervention of advanced technologies such as AI, cloud computing, automation, and real-time data analytics.
In particular, generative AI has represented a substantial technological leap, enabling the implementation of previously unfeasible use cases, especially in customer interaction processes.
Additionally, other AI-based solutions are maturing and helping to automate network operations, such as machine learning, optimization algorithms, natural language processing (NLP), recommendation algorithms, computer vision, and digital twins.
Facilitators and main challenges in the evolution towards autonomous networks
The introduction of advanced technologies can be facilitated by incorporating network computing and software, allowing the integration of intelligent algorithms and providing networks with greater automation and autonomy. Additionally, non-technical factors such as operators' innovation programs and a data-driven culture can accelerate the adoption of hyper-automation solutions.
However, challenges will arise during this process that must be adequately addressed to ensure the success of strategic plans. For instance, the technological maturity level of networks will influence the operator's ability to implement use cases. The lack of an expert team in these solutions or investment capacity can also pose significant challenges.
Another crucial aspect is data management. Without reliable historical data, or if data does not comply with GDPR or is dispersed within the organization, use cases may not yield the expected results. Data quality is essential for the success of AI algorithms in their predictions and recommendations.
Change management also plays a fundamental role. Network hyper-automation transforms essential operational processes and has a significant impact on the personnel operating the network. These individuals will shift from performing tasks to supervising tasks autonomously executed by machines. It will be necessary to accompany this change with communication and reskilling plans to ensure a high level of commitment and alignment throughout the company during the transformation.
In addition, there are inherent risks in introducing autonomous technologies that have been analyzed by the European Commission. To provide a safe regulatory framework, the EU Artificial Intelligence Act was recently published and will be mandatory by 2026. Manufacturers and operators will need to comply with this regulation and adopt measures to minimize risks according to the established classification.
The 6D framework: the comprehensive strategy for AI adoption in autonomous networks
To successfully address the transformation towards autonomous networks, an integrated vision of the process is necessary. At Nae, we have developed a framework called the "6D Framework" to cover the end-to-end of this transformation. This framework includes the following phases:
Discover: establish the degree of implementation of autonomous network solutions.
Design: define the strategy for adopting AI use cases, prioritization, and planning.
Deliver: implement the minimum viable product (MVP) of the defined roadmap.
Develop: develop full functionalities from the MVP and other use cases.
Detect: measure the progress and success of the implemented use cases to feed back into the strategic plan.
Drive: define the program governance and manage change.
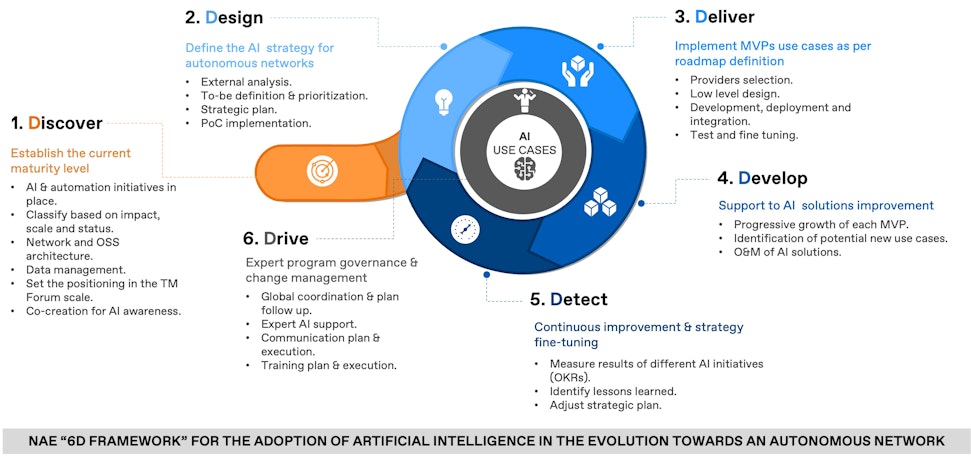
This approach allows for structured and efficient management of the transition towards autonomous networks, ensuring that each phase of the process is properly planned and executed to maximize the impact and sustainability of the implemented solutions.
Later on, we will offer a detailed analysis of this methodology, exploring each phase of the "6D Framework" for AI adoption in the evolution towards an autonomous network.